We often hear poker pundits claim a particular play is the “GTO” play in this situation. For example: “Phil’s AQs is within his 6.2% GTO three-bet range here.” I find such commentary aggravating because it implies that we should be using game theory strategies in our everyday, low-stakes cash games.
Indeed, there are many training sites that purport to do just that. But even if we know the correct Game Theory Optimal (GTO) play, it’s usually not the most profitable play to make. I believe that using GTO strategies in these games is not just a minor mistake; it can be a major profit leak.
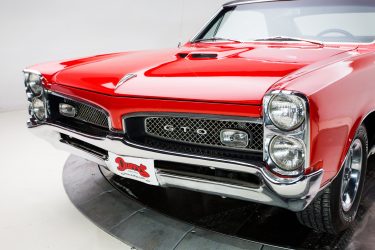
A lot of players these days think GTO is key to adding muscle to their poker game, but sometimes that’s can be a real chip guzzler. (Image: Duffy’s Classic Cars)
“Any fool can have bad luck. The art consists in knowing how to exploit it.”
– Frank Wedekind
First let’s be clear that my GTO criticism applies to no-limit hold ’em (NLH) games that are populated by weak, exploitable players who rarely employ game theory strategies. This certainly includes the smallest, live low-stakes cash games, such as $1/$2 and $2/$5. But it can also include larger Vegas cash games and medium stakes online cash games. It would be rare to face a single GTO player in such a game, let alone multiple GTO players.
Issue 1: Game Theory Not-So-Optimal
A robust field of modern mathematics, game theory initially considered only zero-sum games between two opponents where one person’s gain was the other’s loss. This was eventually expanded to include a wide range of situations. Now it has become a generalized term for the science of decision making, and it is often applied to poker.
One of the simplest game theory applications is for the game Rock-Paper-Scissors (RPS), where rock beats scissors, scissors beats paper, and paper beats rock. I analyzed the math of RPS in Chapter 7.2 of Tournament Poker for the Rest of Us, but it should be obvious that the “optimal” strategy for RPS is for the hero to randomly throw each sign one-third of the time.
Unsurprisingly, this is also his opponent’s “optimal” strategy. Together, these two strategies form what is known as the RPS Nash Equilibrium, named for John Nash of “A Beautiful Mind” fame, who won a Nobel Prize in Economics in 1994 for his work.
Suppose Player A doesn’t know about game theory, and has a playing tendency we can exploit. We have a large number of his RPS hand histories, and we know he throws Rock/Paper/Scissors 40%/35%/25% of the time. We would show a long-term profit against Player A by always throwing paper. Our expectation would be 0.4 – 0.25 = 0.15 betting units. This is called a maximally exploitive strategy, which is what we should use in a short match where he is unlikely to catch on to our strategy.
So if our RPS opponent has a tendency we can exploit, our long-term expectation can be positive. This is true for poker as well as RPS. Since nearly all real poker players make lots of mistakes, we can make more money by exploitive play than by playing perfect a GTO strategy.
Note that if Player A decides to play a GTO strategy against us, our exploitive strategy will not lose in the long run. In fact, as long as one of us uses GTO, our expectation will be zero. This is because the RPS GTO strategy is a mixed strategy, which is a combination of pure strategies. (One pure strategy is to always throw paper, like we might do against Player A.)
Any poker GTO strategy will include many pure strategy decisions, such as always opening with kings or always folding 72o UTG. When our opponent makes pure strategy errors, our GTO play will have a positive expectation against him. Therefore, a GTO strategy will make money in any real poker game where our opponents make lots of errors. This is often used as a rationale for learning and using game theory strategies. We can’t lose with them, but we also won’t maximize our profit with them.
Issue 2: GTO Strategies Based on Toy Games
The RPS game describe above is a simple game played by simple rules. Game theory is much more difficult to apply to real-world poker games. Consequently, early game theory solutions were limited to heads-up limit hold ’em matches. This is a game with only two players, and these players have a small list of options: check, fold, call or raise one unit. So the computational problems are tractable.
This gives us 10 quintillion possibilities to evaluate, not considering the many decisions to be made for each possibility (such as bet sizing or a villain’s range). This is far too many for even the best computers to solve without significant simplification.
So we try to simplify the computation by restricting our game assumptions. For example, we might assume that every player will open with a proper GTO range. We may restrict his opening bet to three BBs. The model may then assume that we three-bet with a GTO range, and that the opener will make a GTO response to our three-bet. We end up with a Nash Equilibrium for this toy game in which neither player is exploitable.
This is rarely the way a real game plays, however. For example, the average open in a $1/$2 Vegas game is five BBs, but it can also be seven BBs, or even 2.5 BBs. Furthermore, the average player only opens about 6.5% of his hands, which is far below a GTO range. So basing our strategy on a particular GTO toy game would be far worse than “optimal.”
Issue 3: Real-Time GTO Difficult to Master
Any GTO solution to a poker situation is based on specific boundary conditions, such as the size of an opening raise or three-bet. This results in a myriad of tables describing our GTO responses, depending on our table position and other considerations. Not only must we properly model this scenario (with a program like PioSolver) or subscribe to a training service that has already solved them, we must also commit each scenario to memory. This is a daunting task.
We must also consider the many different possible boundary conditions that might be applicable to a real-world game situation. For example, we could construct an exploitive GTO fold/call/three-bet strategy based on the opening range of any specific opponent, his typical opening size, his tendency to fold to a specific three-bet size, and so forth. We can also build into our model the propensity of the players behind us to overcall or four-bet. Doing all of this work, and committing it to memory, is nearly impossible for most of us.
Conclusion
I don’t claim that game theory has no value in poker. In fact, Chapter 11 of Tournament Poker for the Rest of Us is largely about the effective use of game theory for short-stack tournament play.
But game theory isn’t very useful for exploitive cash game poker. The time and effort to properly use it in a low-stakes cash game would be better applied to other aspects of poker.